Topic:Deception Detection
What is Deception Detection? Deception detection is the process of identifying deceptive or misleading behavior in text or speech data.
Papers and Code
May 08, 2025
Abstract:Remarkable advancements in generative AI technology have given rise to a spectrum of novel deepfake categories with unprecedented leaps in their realism, and deepfakes are increasingly becoming a nuisance to law enforcement authorities and the general public. In particular, we observe alarming levels of confusion, deception, and loss of faith regarding multimedia content within society caused by face deepfakes, and existing deepfake detectors are struggling to keep up with the pace of improvements in deepfake generation. This is primarily due to their reliance on specific forgery artifacts, which limits their ability to generalise and detect novel deepfake types. To combat the spread of malicious face deepfakes, this paper proposes a new strategy that leverages coarse-to-fine spatial information, semantic information, and their interactions while ensuring feature distinctiveness and reducing the redundancy of the modelled features. A novel feature orthogonality-based disentanglement strategy is introduced to ensure branch-level and cross-branch feature disentanglement, which allows us to integrate multiple feature vectors without adding complexity to the feature space or compromising generalisation. Comprehensive experiments on three public benchmarks: FaceForensics++, Celeb-DF, and the Deepfake Detection Challenge (DFDC) show that these design choices enable the proposed approach to outperform current state-of-the-art methods by 5% on the Celeb-DF dataset and 7% on the DFDC dataset in a cross-dataset evaluation setting.
Via
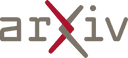
May 05, 2025
Abstract:Gaze may enhance the robustness of lie detectors but remains under-studied. This study evaluated the efficacy of AI models (using fixations, saccades, blinks, and pupil size) for detecting deception in Concealed Information Tests across two datasets. The first, collected with Eyelink 1000, contains gaze data from a computerized experiment where 87 participants revealed, concealed, or faked the value of a previously selected card. The second, collected with Pupil Neon, involved 36 participants performing a similar task but facing an experimenter. XGBoost achieved accuracies up to 74% in a binary classification task (Revealing vs. Concealing) and 49% in a more challenging three-classification task (Revealing vs. Concealing vs. Faking). Feature analysis identified saccade number, duration, amplitude, and maximum pupil size as the most important for deception prediction. These results demonstrate the feasibility of using gaze and AI to enhance lie detectors and encourage future research that may improve on this.
Via
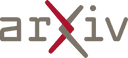
May 01, 2025
Abstract:The proliferation of generative AI and deceptive synthetic media threatens the global information ecosystem, especially across the Global Majority. This report from WITNESS highlights the limitations of current AI detection tools, which often underperform in real-world scenarios due to challenges related to explainability, fairness, accessibility, and contextual relevance. In response, WITNESS introduces the Truly Innovative and Effective AI Detection (TRIED) Benchmark, a new framework for evaluating detection tools based on their real-world impact and capacity for innovation. Drawing on frontline experiences, deceptive AI cases, and global consultations, the report outlines how detection tools must evolve to become truly innovative and relevant by meeting diverse linguistic, cultural, and technological contexts. It offers practical guidance for developers, policy actors, and standards bodies to design accountable, transparent, and user-centered detection solutions, and incorporate sociotechnical considerations into future AI standards, procedures and evaluation frameworks. By adopting the TRIED Benchmark, stakeholders can drive innovation, safeguard public trust, strengthen AI literacy, and contribute to a more resilient global information credibility.
* 33 pages
Via
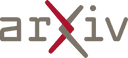
Apr 19, 2025
Abstract:Multimodal fake news detection plays a crucial role in combating online misinformation. Unfortunately, effective detection methods rely on annotated labels and encounter significant performance degradation when domain shifts exist between training (source) and test (target) data. To address the problems, we propose ADOSE, an Active Domain Adaptation (ADA) framework for multimodal fake news detection which actively annotates a small subset of target samples to improve detection performance. To identify various deceptive patterns in cross-domain settings, we design multiple expert classifiers to learn dependencies across different modalities. These classifiers specifically target the distinct deception patterns exhibited in fake news, where two unimodal classifiers capture knowledge errors within individual modalities while one cross-modal classifier identifies semantic inconsistencies between text and images. To reduce annotation costs from the target domain, we propose a least-disagree uncertainty selector with a diversity calculator for selecting the most informative samples. The selector leverages prediction disagreement before and after perturbations by multiple classifiers as an indicator of uncertain samples, whose deceptive patterns deviate most from source domains. It further incorporates diversity scores derived from multi-view features to ensure the chosen samples achieve maximal coverage of target domain features. The extensive experiments on multiple datasets show that ADOSE outperforms existing ADA methods by 2.72\% $\sim$ 14.02\%, indicating the superiority of our model.
Via
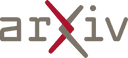
Apr 10, 2025
Abstract:We demonstrate how AI agents can coordinate to deceive oversight systems using automated interpretability of neural networks. Using sparse autoencoders (SAEs) as our experimental framework, we show that language models (Llama, DeepSeek R1, and Claude 3.7 Sonnet) can generate deceptive explanations that evade detection. Our agents employ steganographic methods to hide information in seemingly innocent explanations, successfully fooling oversight models while achieving explanation quality comparable to reference labels. We further find that models can scheme to develop deceptive strategies when they believe the detection of harmful features might lead to negative consequences for themselves. All tested LLM agents were capable of deceiving the overseer while achieving high interpretability scores comparable to those of reference labels. We conclude by proposing mitigation strategies, emphasizing the critical need for robust understanding and defenses against deception.
Via
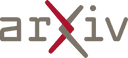
Apr 05, 2025
Abstract:Studying deception in AI agents is important and difficult due to the lack of model organisms and sandboxes that elicit the behavior without asking the model to act under specific conditions or inserting intentional backdoors. Extending upon $\textit{AmongAgents}$, a text-based social-deduction game environment, we aim to fix this by introducing Among Us as a rich sandbox where LLM-agents exhibit human-style deception naturally while they think, speak, and act with other agents or humans. We introduce Deception ELO as an unbounded measure of deceptive capability, suggesting that frontier models win more because they're better at deception, not at detecting it. We evaluate the effectiveness of AI safety techniques (LLM-monitoring of outputs, linear probes on various datasets, and sparse autoencoders) for detecting lying and deception in Among Us, and find that they generalize very well out-of-distribution. We open-source our sandbox as a benchmark for future alignment research and hope that this is a good testbed to improve safety techniques to detect and remove agentically-motivated deception, and to anticipate deceptive abilities in LLMs.
* 17 pages, preprint
Via
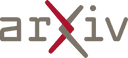
Apr 10, 2025
Abstract:News media, particularly video-based platforms, have become deeply embedded in daily life, concurrently amplifying risks of misinformation dissemination. Consequently, multimodal fake news detection has garnered significant research attention. However, existing datasets predominantly comprise user-generated videos characterized by crude editing and limited public engagement, whereas professionally crafted fake news videos disseminated by media outlets often politically or virally motivated pose substantially greater societal harm. To address this gap, we construct FMNV, a novel dataset exclusively composed of news videos published by media organizations. Through empirical analysis of existing datasets and our curated collection, we categorize fake news videos into four distinct types. Building upon this taxonomy, we employ Large Language Models (LLMs) to automatically generate deceptive content by manipulating authentic media-published news videos. Furthermore, we propose FMNVD, a baseline model featuring a dual-stream architecture integrating CLIP and Faster R-CNN for video feature extraction, enhanced by co-attention mechanisms for feature refinement and multimodal aggregation. Comparative experiments demonstrate both the generalization capability of FMNV across multiple baselines and the superior detection efficacy of FMNVD. This work establishes critical benchmarks for detecting high-impact fake news in media ecosystems while advancing methodologies for cross-modal inconsistency analysis.
Via
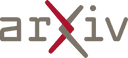
Mar 31, 2025
Abstract:The rapid and unrestrained advancement of generative artificial intelligence (AI) presents a double-edged sword: while enabling unprecedented creativity, it also facilitates the generation of highly convincing deceptive content, undermining societal trust. As image generation techniques become increasingly sophisticated, detecting synthetic images is no longer just a binary task: it necessitates interpretable, context-aware methodologies that enhance trustworthiness and transparency. However, existing detection models primarily focus on classification, offering limited explanatory insights into image authenticity. In this work, we propose FakeScope, an expert multimodal model (LMM) tailored for AI-generated image forensics, which not only identifies AI-synthetic images with high accuracy but also provides rich, interpretable, and query-driven forensic insights. We first construct FakeChain dataset that contains linguistic authenticity reasoning based on visual trace evidence, developed through a novel human-machine collaborative framework. Building upon it, we further present FakeInstruct, the largest multimodal instruction tuning dataset containing 2 million visual instructions tailored to enhance forensic awareness in LMMs. FakeScope achieves state-of-the-art performance in both closed-ended and open-ended forensic scenarios. It can distinguish synthetic images with high accuracy while offering coherent and insightful explanations, free-form discussions on fine-grained forgery attributes, and actionable enhancement strategies. Notably, despite being trained exclusively on qualitative hard labels, FakeScope demonstrates remarkable zero-shot quantitative capability on detection, enabled by our proposed token-based probability estimation strategy. Furthermore, FakeScope exhibits strong generalization and in-the-wild ability, ensuring its applicability in real-world scenarios.
Via
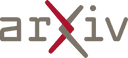
Mar 28, 2025
Abstract:Typosquatting is a long-standing cyber threat that exploits human error in typing URLs to deceive users, distribute malware, and conduct phishing attacks. With the proliferation of domain names and new Top-Level Domains (TLDs), typosquatting techniques have grown more sophisticated, posing significant risks to individuals, businesses, and national cybersecurity infrastructure. Traditional detection methods primarily focus on well-known impersonation patterns, leaving gaps in identifying more complex attacks. This study introduces a novel approach leveraging large language models (LLMs) to enhance typosquatting detection. By training an LLM on character-level transformations and pattern-based heuristics rather than domain-specific data, a more adaptable and resilient detection mechanism develops. Experimental results indicate that the Phi-4 14B model outperformed other tested models when properly fine tuned achieving a 98% accuracy rate with only a few thousand training samples. This research highlights the potential of LLMs in cybersecurity applications, specifically in mitigating domain-based deception tactics, and provides insights into optimizing machine learning strategies for threat detection.
* 6 pages, 1 figure
Via
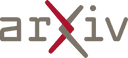
Mar 20, 2025
Abstract:This paper presents the Deceptive Humor Dataset (DHD), a novel resource for studying humor derived from fabricated claims and misinformation. In an era of rampant misinformation, understanding how humor intertwines with deception is essential. DHD consists of humor-infused comments generated from false narratives, incorporating fabricated claims and manipulated information using the ChatGPT-4o model. Each instance is labeled with a Satire Level, ranging from 1 for subtle satire to 3 for high-level satire and classified into five distinct Humor Categories: Dark Humor, Irony, Social Commentary, Wordplay, and Absurdity. The dataset spans multiple languages including English, Telugu, Hindi, Kannada, Tamil, and their code-mixed variants (Te-En, Hi-En, Ka-En, Ta-En), making it a valuable multilingual benchmark. By introducing DHD, we establish a structured foundation for analyzing humor in deceptive contexts, paving the way for a new research direction that explores how humor not only interacts with misinformation but also influences its perception and spread. We establish strong baselines for the proposed dataset, providing a foundation for future research to benchmark and advance deceptive humor detection models.
* 15 Pages, 4 figures, 8 tables
Via
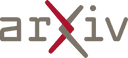